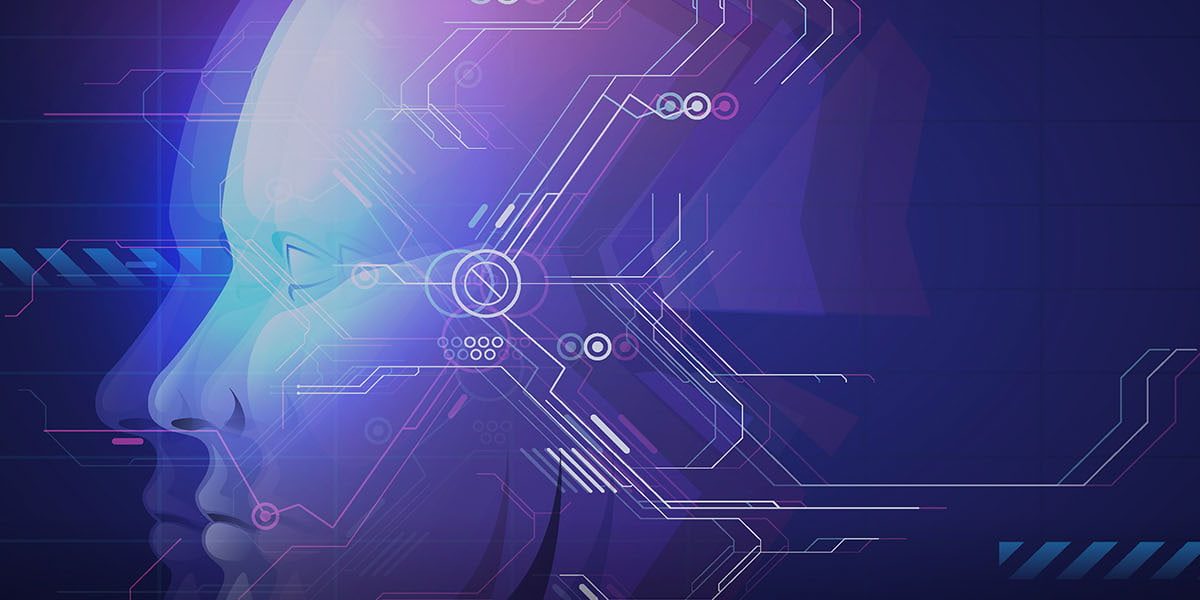
AI TRiSM: trust, risk and security management for Artificial Intelligence
Artificial Intelligence (AI) has become a crucial component for innovation and operational efficiency. However, with its growing adoption, significant challenges related to trust, risk, and security have emerged. This is where AI TRiSM (Artificial Intelligence Trust, Risk, and Security Management) comes into play, offering a comprehensive approach that advocates for the creation of reliable, secure AI systems capable of managing risks effectively.
AI TRiSM originated from the pressing need to address the concerns inherent in implementing AI systems. As organizations began integrating AI into their operations, it became clear that, although powerful, these models are not without flaws and vulnerabilities. Why? Let's break it down.
AI vs. Reality
In the context of implementing AI systems, trust is a critical aspect that must be addressed with technical rigor. Trust issues primarily arise from algorithm opacity, inherent bias in training data, and the lack of explainability in models.
Algorithm opacity refers to the difficulty of understanding how and why an AI model makes certain decisions, which can lead to distrust from both users and regulators. Bias in data, which can result from non-representative or prejudiced information gathering, leads to discriminatory and inequitable outcomes, negatively affecting specific groups of people. Lack of explainability, on the other hand, prevents stakeholders from understanding and validating the model's decisions, limiting their ability to intervene in case of unexpected or erroneous results.
In Latin America, a notable example illustrates the magnitude of these challenges, with a case regarding the implementation of a crime prediction system: In 2019, Argentina developed an AI system to predict areas and times with a high probability of crimes. However, the system faced significant criticism due to the lack of transparency in its algorithms and the bias in the data used, which resulted in disproportionate surveillance in neighborhoods with large concentrations of disadvantaged communities. This not only generated distrust among citizens but also raised serious security and privacy concerns. The opacity of the system prevented citizens from understanding the criteria for surveillance, while the data bias reinforced stereotypes and perpetuated discriminatory practices.
Breaking Barriers!
Despite the challenges related to trust, risk, and security, the proper implementation of AI models through AI TRiSM can radically transform these issues into opportunities. By adopting transparency and explainability practices, organizations can build AI systems that are not only understandable but also auditable. This means that users and regulators can clearly see how and why AI makes certain decisions, which increases trust in these systems. Transparency helps identify and correct potential biases and errors before they cause significant problems. By making algorithms easy to understand, organizations not only strengthen trust in their technologies but also facilitate collaboration and regulatory compliance, turning initial challenges into competitive advantages and innovation opportunities.
Additionally, proactive risk management and the implementation of robust security measures are essential components of AI TRiSM that can prevent potential threats and protect the integrity of AI systems. By conducting regular risk assessments and applying advanced cybersecurity techniques, organizations can identify and mitigate vulnerabilities before they are exploited. This not only reduces the risk of adversarial attacks but also ensures that AI systems operate consistently and reliably.
We must say that the benefits of well-managed AI through AI TRiSM extend beyond mere risk mitigation. The ability to create reliable and secure AI systems allows organizations to innovate with confidence, harnessing the full potential of AI to improve operational efficiency, optimize decision-making, and provide superior user experiences.
For example, in the financial sector in Latin America, the use of AI for fraud detection and credit risk assessment has become more accurate and efficient thanks to the implementation of AI TRiSM principles. By ensuring transparency, security, and risk management, financial institutions can offer safer and more personalized services, increasing customer satisfaction and strengthening their competitive position in the market.
TOP 3 Key Trends
1. Transparency and Explainability: For AI systems to build trust, it is crucial that they are clear and understandable. AI models must be easy to interpret, allowing users to understand how decisions are made. This not only increases trust in the system but also helps identify and correct potential biases and errors. Transparency ensures that all stakeholders, from developers to end users, can assess the internal workings of the system, facilitating the detection of failures and continuous model improvement. Explainability means that AI decisions can be explained in a clear and logical manner, which is essential for the adoption and acceptance of these systems in critical areas like healthcare, justice, and finance.
2. Risk Management in AI: It is important to identify, assess, and mitigate risks when implementing AI systems. This includes ensuring that the model is robust, that the data is accurate, and that the system is protected against potential attacks. Effective risk management also involves constant monitoring of AI systems to detect and quickly mitigate any emerging threats. This proactive approach not only protects the system's integrity but also ensures its operational continuity in adverse scenarios. Moreover, risk management should include contingency and recovery plans to ensure that any disruption can be handled efficiently with minimal impact on the organization.
3. Data Privacy and Security: Data privacy is crucial when using AI systems. These systems must comply with privacy laws and ensure that personal information is handled securely and ethically. This involves protecting sensitive user data and ensuring it is not misused. Additionally, it is essential to implement cybersecurity measures to protect AI systems from attacks that could compromise their integrity or functionality. This includes preventing unauthorized access and malicious tampering, ensuring that AI models operate reliably and securely. The combination of privacy and security not only protects users but also strengthens trust in AI systems, promoting their adoption and responsible use across industries.
Modalities for Optimal Implementation
To achieve an effective AI TRiSM model, it is essential to combine computer, human, and operational modalities in an integrated and coherent manner. Computer modalities focus on implementing tools and techniques that ensure fairness, transparency, and security in AI systems. For example, algorithmic auditing is essential for reviewing and validating AI algorithms, ensuring they operate fairly and without bias. Additionally, continuous monitoring enables real-time anomaly detection and quick response to potential security incidents, ensuring that systems are robust and reliable. Data encryption and anonymization are also crucial for protecting the privacy of the data used and generated by AI systems, preventing the misuse of sensitive information.
In parallel, human modalities play a key role in implementing AI TRiSM. Employee training and awareness of security and ethical practices in AI are vital for understanding risks and adopting the best practices associated with them. Well-trained employees can identify and mitigate potential problems before they become serious threats. Furthermore, human oversight in the AI system lifecycle ensures that critical decisions are reviewed by experts, which is essential for maintaining trust and accountability in AI use.
Operational modalities complement this approach by developing and maintaining clear policies and procedures for managing security and risk in AI, aligned with industry regulations and standards. The existence of well-defined policies ensures that all security practices are consistent and effective throughout the organization. Conducting periodic risk assessments is also essential for identifying new threats and proactively updating mitigation strategies. This dynamic approach ensures that organizations can quickly adapt to an ever-evolving threat environment.
In summary, the combination of computer, human, and operational modalities is crucial for building an effective AI TRiSM model. Each of these modalities brings unique elements that, when integrated, enable organizations not only to protect themselves against threats but also to safely and ethically leverage the benefits of artificial intelligence.
To conclude, it is important to recap that AI TRiSM, as a comprehensive approach to addressing the challenges associated with AI implementation, enables organizations to create reliable and robust AI systems. At Novacomp, we understand the importance of these models and are committed to providing highly trained teams that not only drive innovation but also protect the integrity and privacy of your data through a combination of computer, human, and operational modalities. We can help your organization navigate the challenging AI landscape safely and efficiently!